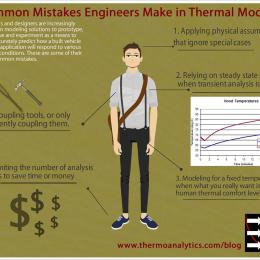
Engineers and designers increasingly rely on modeling solutions to prototype, test, prove and experiment to more accurately predict how a built vehicle or other application will respond to various thermal conditions. While these methods are designed and promoted to save manufacturers time and money, five common mistakes made during the modeling phase can actually cost you more money, add time to the design cycle, and ultimately hurt product performance.
Here are the five most common mistakes and how you can avoid them:
1.) Relying on steady-state analysis when the transient analysis is required.
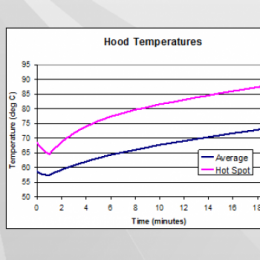
It is a natural and seemingly intuitive temptation to simplify the test scenario to save costs or mitigate modeling complexity. In doing so, many mistakenly model their testing environment as an “equilibrium” or “steady state.”
But the real world is anything but “steady.” We live in a transient world where a car's performance (for example) is influenced by its ability to respond to dynamic forces.
- Sometimes we drive short distances, with many starts and stops; other times, we drive long distances at a fairly consistent speed.
- Sometimes we tow a heavy load uphill; other times, we coast downhill.
- Sometimes we drive at night when it’s cooled down outside; other times, we run quick errands during the hottest part of the day.
To replicate an automobile's real-world conditions, it is important to use a “transient analysis” tool that can best model reality. Otherwise, you end up over-designing and over-manufacturing. You potentially end up with an HVAC system that is more powerful (read: more costly and less energy-efficient) than necessary, or you add unnecessary insulation or shielding — adding design, manufacturing, and material costs.
2.) Limiting the number of analysis cases to save time or money.
Engineers will often limit the variables they are accounting for when thermal modeling is a way to save costs in design. In exchange, a tradeoff might be to account for the “worst-case” scenarios, hoping that one is accounting for the broadest range of likely outcomes. But often, one’s assumptions turn out to be counterintuitive.
For example, you might test a high-performance vehicle at high speeds to see how it performs under strenuous conditions. However, in this case, high speed might not be the most strenuous of circumstances, as there is also a high volume of airflow when a car travels at high speeds, which can cool an engine.
This again leads to either over-designing or under-designing, as you’re practically guessing at conditions based on intuition rather than simulating and testing against real-world scenarios. Running a high-fidelity model with a fast analysis tool will allow you to account for all variables and test cases, without which you could miss a potential problem you will find later in the design cycle. At this point, changes are more expensive and detrimental to the overall project lifecycle, so you save neither time nor money after all.
3.) Applying physical assumptions that ignore special cases.
Assumptions in testing environments can be useful and potentially time-saving, but they can also be risky and inaccurate — especially if you don’t account for special cases. An illustrative example is a certain hotel-casino in Las Vegas. The design called for a windowed, curved façade. However, the design was likely never thermally modeled in its real-world environment, and the assumptions proved costly.
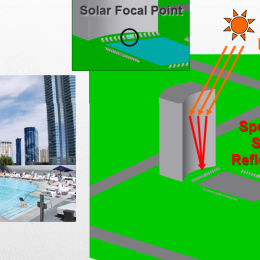
On the concave side of the building, the sun’s rays were concentrated at certain times of the day, making specific areas in front of the hotel uncomfortably hot for guests and making that part of the hotel unusable for part of the day. Had this been fully modeled to account for the special times of day at which the curvature of the glass and intensity of the sun’s heat combined to create a “focusing” effect of the solar load, this special-case scenario could’ve been predicted … and, more importantly, avoided. Instead, this hotel has achieved the unfortunate moniker of “The Death Ray Hotel.”
We see this in building design quite frequently, but it’s also prevalent in other applications as well, such as in solar shields or awnings that have reflective (“specular”) surfaces that can bounce light and heat in utterly unpredictable ways — unless, of course, you accurately model it and test it.
4.) Modeling for a fixed temperature level when you want a desired human thermal comfort level.
It’s one thing to test an automotive HVAC system’s ability to achieve a “climate control” of 72 degrees … quite another to verify whether the human in that cabin is actually comfortable. The Berkley Comfort Model explicitly accounts for physiology-based metrics in predicting passenger comfort, which measures and accounts for the physiological response to environmental factors, such as metabolic heating, shivering, respiration, blood vessel dilation or constriction, perspiration, clothing factors, and even the passenger’s activity level just before entering the vehicle.
In fact, a recent study showed that time to comfort could vary by almost a factor of two, depending on a passenger’s pre-cool-down activity, which alone demonstrates the disparity between measuring environmental metrics only as opposed to physiology-based comfort metrics.
Failing to test for human comfort, as opposed to simple climate control
can potentially lead to higher energy operating costs or to a design that doesn’t have the desired effect of making the passenger comfortable in a reliable, efficient manner.
5.) Not coupling tools or only insufficiently coupling them.
The engineering community is beginning to realize that coupling a thermal analysis solution with a CFD tool is a more reliable way to model thermal dynamics. Through co-simulation of thermal and CFD, you can account for greater variations.
But keep in mind current CFD tools are too computationally intensive for transient states, so they can return imprecise thermal results in a standalone mode. Under the hood of an automobile, for example, you have three different factors affecting heat transfer — radiation from the engine itself, conduction of heat through parts in contact with one another, and convection caused by inconsistent airflow — that all vary in degree and duration throughout the real-life drive cycle.
As a result, knowing when to couple solutions is equally important as knowing which tools to the couple. Coupling early in the design cycle allows you to perform one test and use the results to establish the boundary conditions for the next test. Continuing that process throughout the design cycle results in “virtual prototyping” — modeling and simulation that is done early in the design phase, with the potential to dramatically reduce concept-to-completion times … and realize significant financial savings as a result.
Want to Avoid These Mistakes?
How do you avoid these mistakes? Perform transient analysis case studies with a high-fidelity, fast solver.
Traditional, “steady state” analysis operates like a “pristine” testing laboratory that provides some useful data but also misses many of the elements that exist in the real world. Transient analysis more closely replicates, models, and predicts real-world scenarios and real-life outcomes.
And that means lower production costs, faster and more precise design, and better performance for your customers.
Learn more about our transient thermal simulation software, TAITherm.
Visit our website at suppport.thermoanalytics.com for
- FAQs
- Webinars
- Tutorials
Get help from our technical support team: